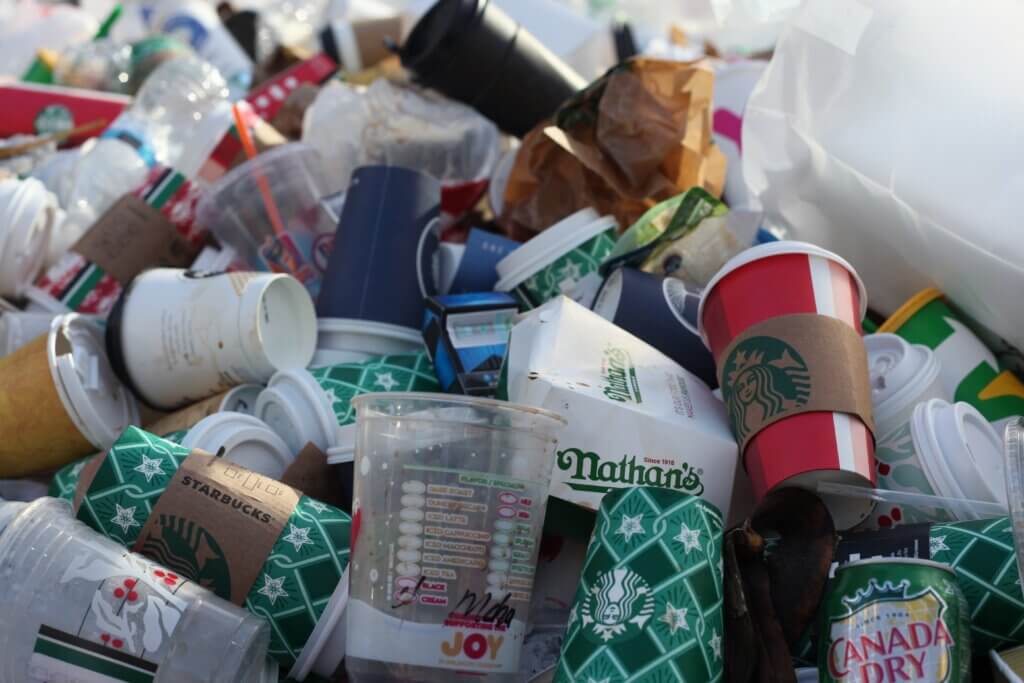
All manufacturers naturally produce waste.
Food manufacturing is no exception. As I explained in this post, surplus production and food spoilage result in many tons of wasted food and energy every year.
Yet in the push for more efficiency and throughput, lean manufacturing requires that we focus on eliminating waste (not just scrap). Doing so will automatically reduce cost, while naturally increasing volume and sustainability in the process. A ‘win-win’ for the industry and the environment.
Achieving this level of efficiency will require advanced analytics to predict demand accurately, adjust inventory levels optimally, and fine-tune production runs accordingly.
The big question is, “How?”
Let’s explore how Data Science can be applied to 5 key areas of food manufacturing to improve sustainability.
1. Food Waste
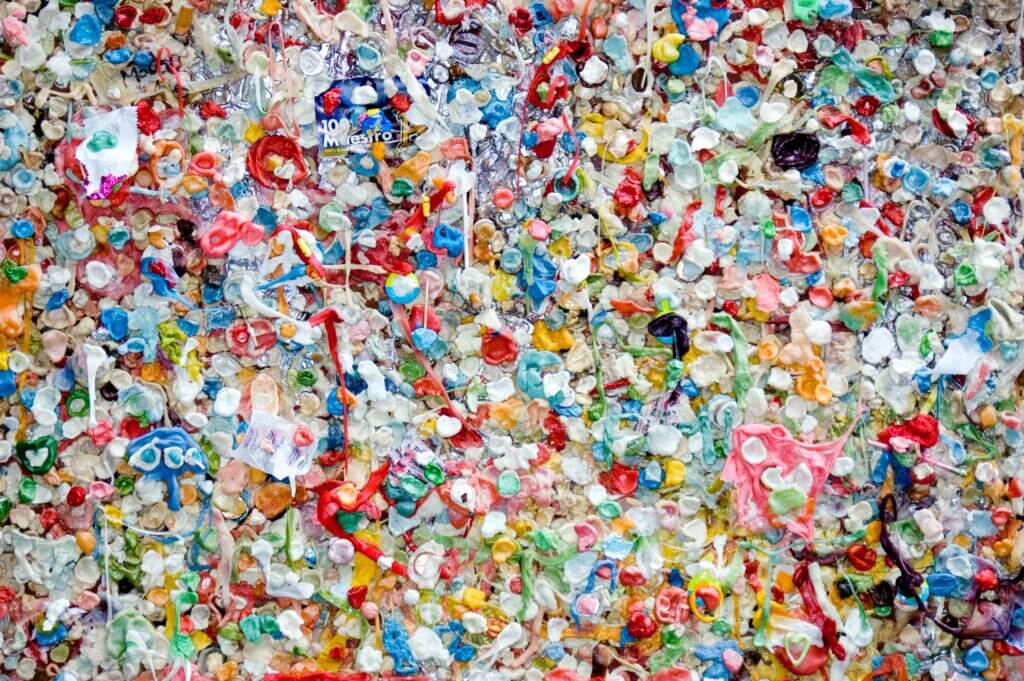
The first place to look into waste reduction is food waste itself.
Nearly a third of the food produced every year is wasted. With it, so is the water, soil nutrients, transportation energy, and labor that went into producing it.
In food manufacturing, inefficiency in the production process is the leading cause of food waste.
Quality defects, such as variances in weight, shape, and appearance are a common cause of scrap. Because food quality is process-driven, the causes of scrap are often hidden from view.
Poor quality in food manufacturing is mostly a mathematical issue that can now be solved thanks to developments in artificial intelligence and advanced analytics.
How Data Science Can Help
- AI-powered Optical Sorting systems can identify defects in food 20,000x faster than human inspectors, and the data generated can be used to build Machine Learning algorithms to fine-tune production lines upstream to drive more stringent quality control.
- Model-based inventory control: applying probabilistic models to historical data to recommend the ideal inventory levels.
- Demand forecasting models can help avoid surplus production and food spoilage.
- Using sensor data to build predictive maintenance models and avoid unplanned downtime and scrap.
2. Energy Consumption
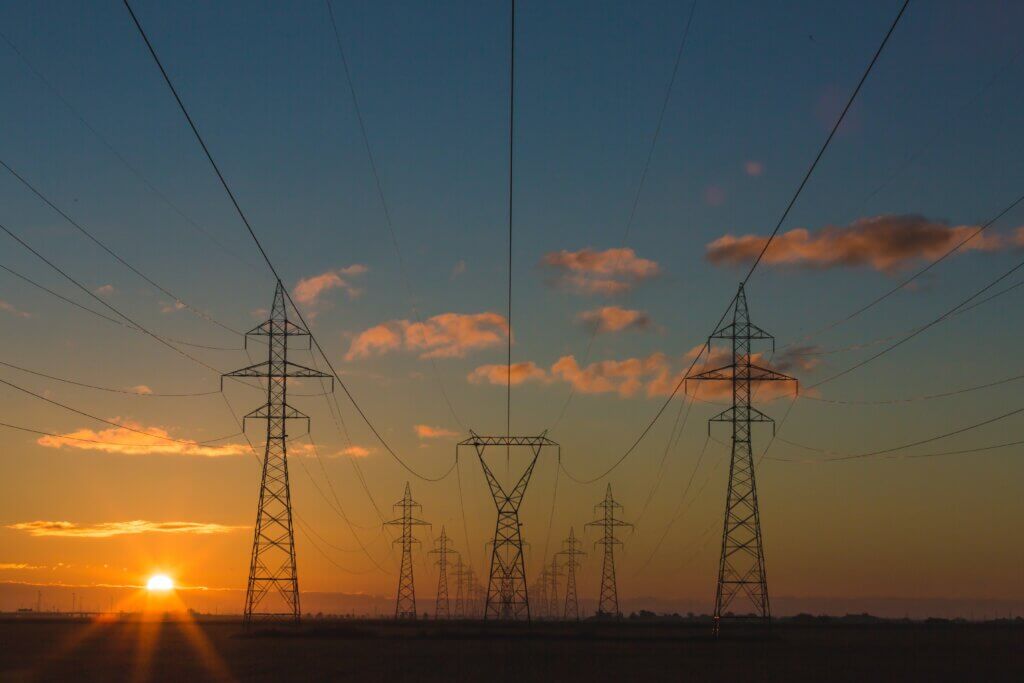
Food manufacturing and processing are energy-intensive industries.
Energy conservation not only makes economic sense, it can have a big impact on a manufacturer’s sustainability goals. Furthermore, continuous improvements in plant energy efficiency can help reduce GHG emissions.
Combining smart power metering systems with data science can highlight energy consumption patterns and optimal control for individual machines, production lines, or even entire factories.
How Data Science Can Help
- Measuring energy consumption from chillers, air-compressors, boilers and other auxiliary systems in food manufacturing can be used to model and predict energy used.
- HVAC performance simulations and ambient weather data can be combined with Deep Learning to predict thermal loads in factory plants.
- Using low-cost IIoT sensors for anomaly detection in cold storage can be used to measure refrigeration energy performance and alert when any issues arise, thereby preventing spoilage.
- Energy forecasting models can be used to identify times of peak energy demand and adjust consumption accordingly.
3. Packaging
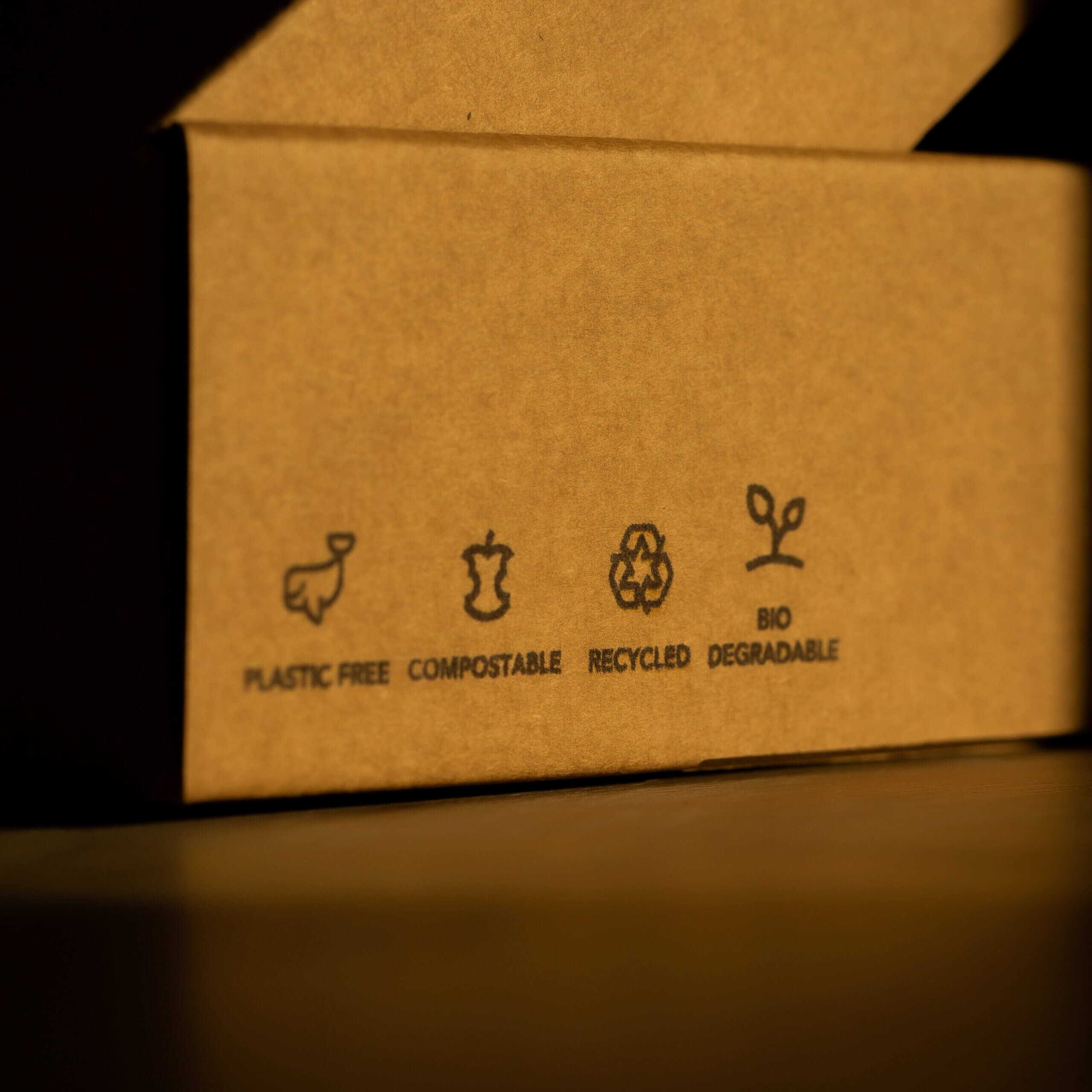
Product packaging is important in every industry, but nowhere is it more important than for the safe delivery and storage of food.
However, as plastics from food packaging continue to clog our landfills and our oceans, it is now critical that we look for ways to package our foods more sustainably. A great place to learn more about advances in this space is Cory Conner’s excellent Sustainable Packaging Podcast.
Advanced analytics are helping food manufacturers look for ways to safely and sustainably package the food we eat.
How Data Science Can Help
- Use historical data from packing machines to assess the efficiency of their process and look for ways to reduce packaging scrap.
- Advanced analytics is being used to design compostable packaging, and new materials that help keep food fresher longer. For example, bags that keep bread from molding.
- Smart labels, RFID (radio-frequency identification), NFC (near-field communication), and Bluetooth are some examples of the sensors on packaging that may interact dynamically with intelligent packaging systems by utilizing the Internet of Things and Big Data, enabling businesses to make informed decisions quickly.
- By merging sensor data, supply chain, custody, and big data analytics to trace food from source to store, there have been significant advancements in food safety.
4. Supply Chains
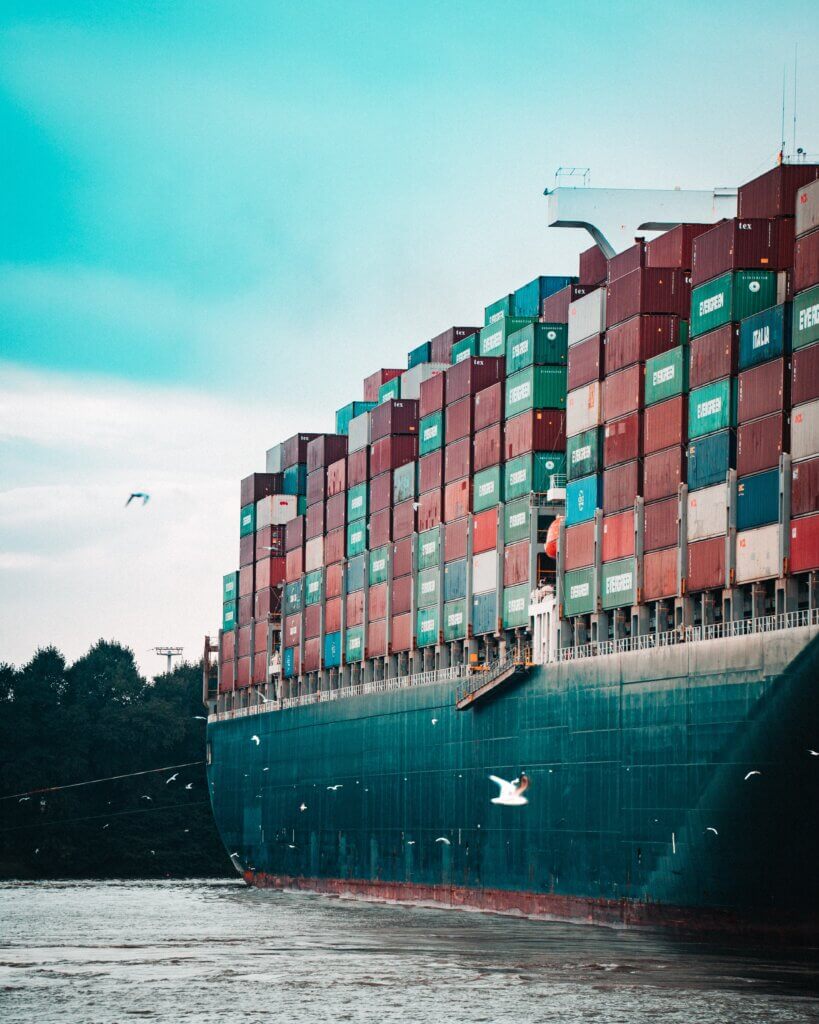
Recent disruptions have shown how vulnerable supply chains are for food manufacturers.
From sourcing ingredients, to finding spare parts for equipment, every food & beverage company has felt the pain from inefficient supply chains. Compounding this pain are increasing demands from consumers for next-day delivery, the dreaded “Bullwhip effect,” and the risk of stale inventory, storage costs, and excess food and energy waste.
Supply chain analytics allows manufacturers to collect, evaluate, and take action on the data produced by their internal operations and supply network to ensure greater robustness and efficiency in their supply chains.
How Data Science Can Help
- Supply-chain forecasting: the act of determining, with data, when suppliers will have a product ready & at what point it should be ordered.
- Supply-chain optimization: Use of Linear Programming Models, such as Monte Carlo simulations, to find the optimal combination of suppliers and distribution centers.
- Transportation route optimization: organize delivery routes and truck loading to reduce wasted fuel and costs.
- Inventory management: reduce ordering, storage, and shortage costs while taking the demand distribution into account.
5. Sustainable Ingredients
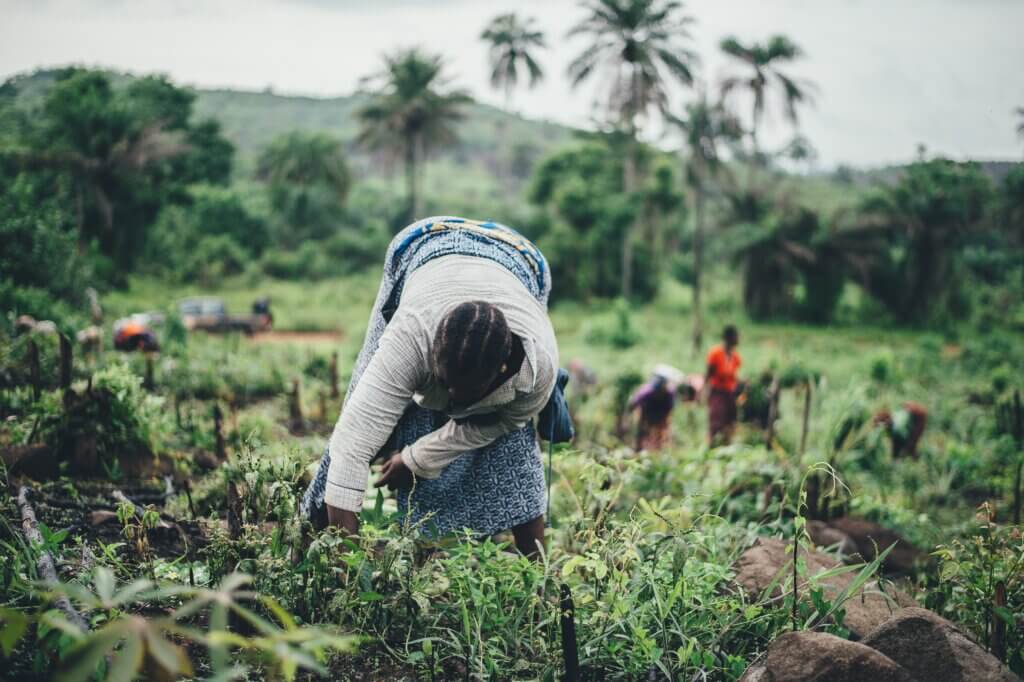
Quality ingredients make all the difference in food manufacturing.
Not all ingredients are created equal, however.
Particularly with regard to agriculture, there are a variety of agricultural products whose production raises questions about social and environmental sustainability. For example, the cultivation of “dirty” commodities like palm oil, cocoa, and coffee has been connected to deforestation, habitat loss, child labor, and forced labor, among other sustainability concerns.
Data Science can be used to examine sustainability and sourcing in the food supply chain.
How Data Science Can Help
- Data Science can enhance supply-chain visibility by automating and analyzing supplier data to extract key sustainability metrics and certifications.
- Automatically analyze sourcing data to flag questionable products and ingredients.
- Analyze, report, and predict CO2 emissions in your distribution network.
- Optimize transportation routes and truck loading to minimize cost and emissions.
All of these steps, together, is what creates more sustainable food manufacturing.
Once you have the data, there are ways to use it to maximize opportunities in sustainability and waste (not just scrap) reduction — and it’s called Data Science.