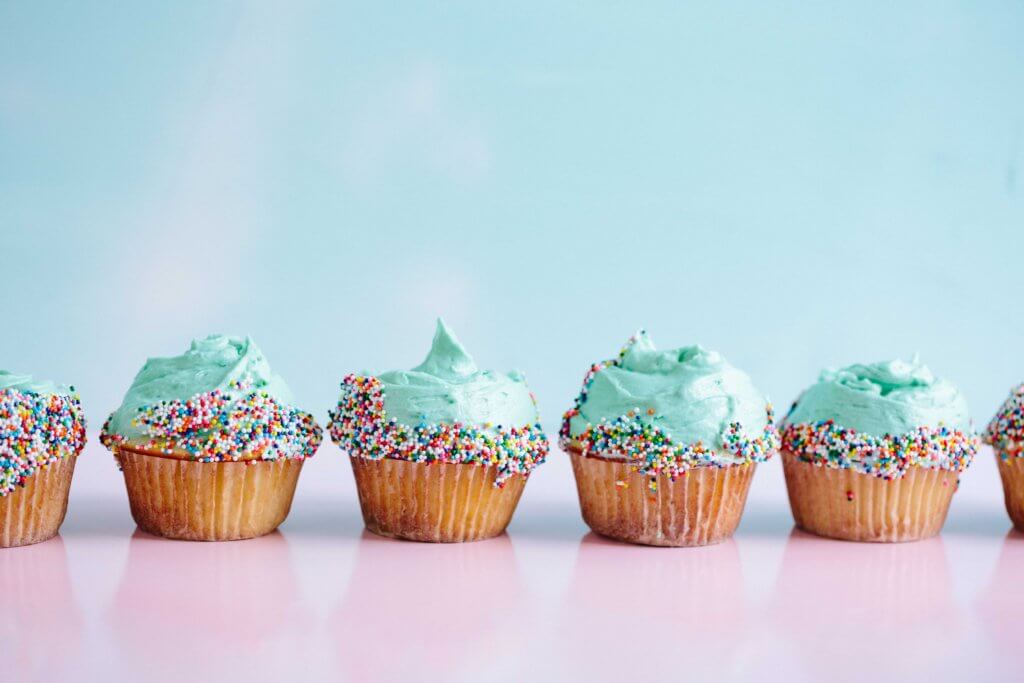
An Introduction to DIY No-Code Demand Forecasting for Food
Photo by Brooke Lark on Unsplash
The Series…
Learn How to Easily Build Your Own Demand Forecasting Model With No-Code.
Recently, I sent a series of polls to various food groups on LinkedIn to ask what topics they were most interested in learning with-respect-to data science in food manufacturing. AI & predictive analytics consistently came out on top.
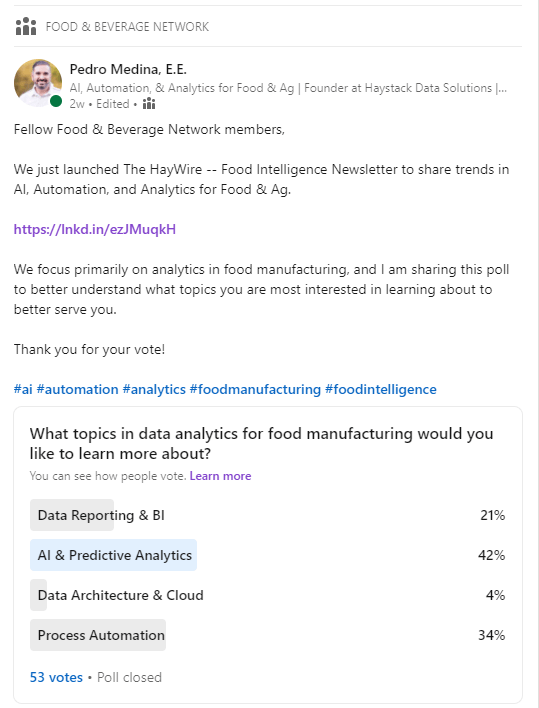
Based on the results of the polls, and the success of a recent demand forecasting project for a commercial baking customer, I am writing a four part series to show how food manufacturers can improve demand prediction.
Every food manufacturer can accurately predict product demand by designing and developing their own forecasting models using free, open-source no-code analytic tools.
The goal of the series is to gently walk you through every step you need to easily blend, transform, visualize, and finally, model your historical product data to accurately predict future product performance.
Using a combination of statistical techniques (Don’t worry, we’ll focus more on the concepts and less on the math), such as ARIMA models, exponential smoothing, and Recurrent Neural Networks, I will show you how easy it is to build sophisticated solutions using the free, KNIME no-code analytics platform.
The series will be broken down as follows:
Part 1. An Introduction to Demand Forecasting for Food Manufacturing
Part 2. Getting Started: Load, Clean, & Explore Your Data
Part 3. Classical Forecasting Techniques
Part 4. Introduction to Machine Learning Based Models
➼ By the end of the series, you will be able confidently build your own demand forecast model, seamlessly integrate your current data sources, and generate insights in minutes without the need to invest in expensive 3rd party products.
But first, why is demand forecasting so important for food manufacturers? To learn why, let’s see how a commercial baker dealt with the challenge of predicting demand for their perishable products.
The Context…
The challenge of predicting demand for perishable baked goods.
Not long ago, one of our customers – a mid-sized commercial bakery in the Midwest – was struggling with forecasting demand for their products. With over 200 product SKUs, this was no easy feat!
They had no formal forecasting process in place – just spreadsheets they would email to managers and salespeople in order to gather feedback based on their current knowledge of market trends. A demand manager would then perform a simple moving average of sales for the prior 4 weeks for all products combined, and proceed to manually stitch spreadsheets together as his team’s input slowly trickled in, adjusting his numbers as he went.
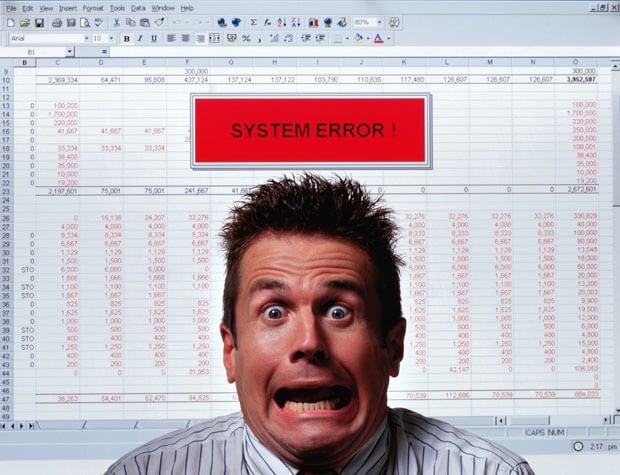
Eventually, he would share his tenuous forecast with senior leaders who would then agonize over its accuracy in supporting their deep operational and strategic decisions.
Inevitably, this simple and time-consuming planning process would result in a weekly scramble as orders would not come in as expected.
It goes without saying, then, that accurate demand forecasting is essential to efficient and profitable food manufacturing. However, commercial bakers are especially affected given the perishable nature of their products.
The short shelf-life of baked goods – typically 1 to 3 weeks – makes having the right product at the right amount crucial to a baker’s success.
The sheer quantity of SKUs is another challenge for commercial bakers. Scheduling production runs for so many different products is complex. For instance, not every product can be manufactured every week due to constraints around allergens, recipe mixes, and the ongoing scarcity of ingredients and price inflation. What’s more, our customer’s production schedule was on a fixed 2-week run, which required even more accurate projections.
With no way to accurately predict demand for their products, their revenue, cost, and pricing forecasts were often just as unreliable. Nor could they flexibly react to seasonal demand swings that they couldn’t see coming.
Additionally, our commercial baker would introduce new products to the market several times a year. Previously, they relied solely on “gut instinct” or cursory market research to anticipate how these new products might perform with consumers.
➼ Despite sitting on a gold mine of historical product data, their hopes were often dashed (along with significant investments in R&D) when new products flopped or underperformed simply because they didn’t know how to leverage their data to tease insights into future market performance.
Furthermore, they had no way of calculating the cannibalization effects new products have on existing ones. Consequently, they couldn’t measure just how much they were robbing Peter to pay Paul, pastry products notwithstanding.
With so much at stake, the case for building an accurate forecasting solution was obvious.
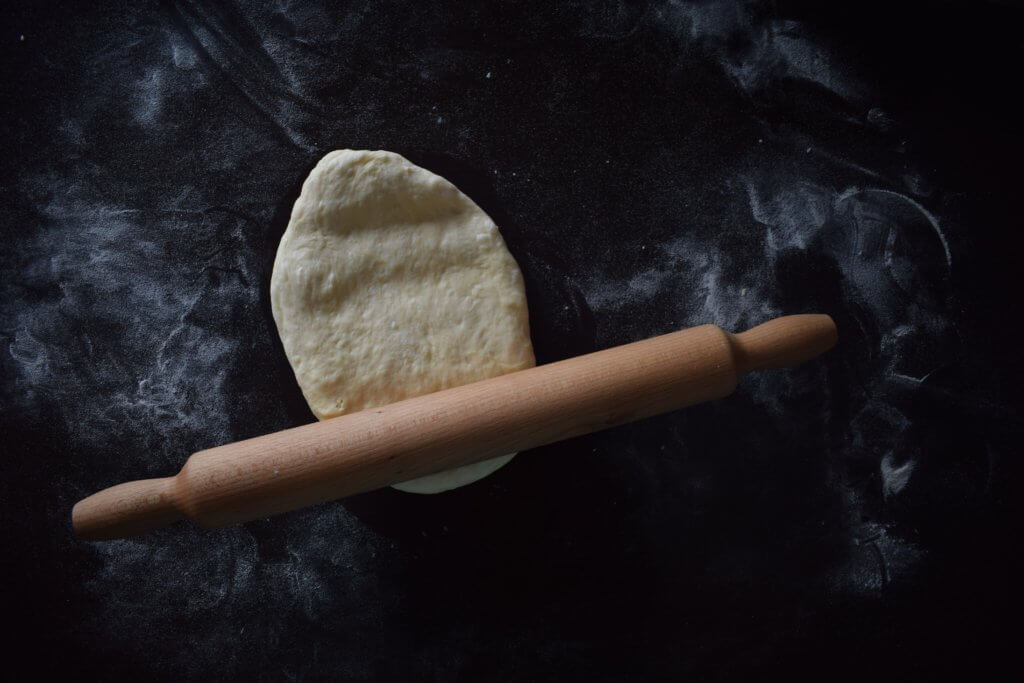
A Free & Flexible Forecast
Photo by Tamara Gak on Unsplash
The Solution…
Generate Forecasts for New and Existing Products in Minutes.
Fortunately, our customer saw the glaring need to improve product demand forecasts for their production planning. But, they didn’t know where to begin.
They knew they wanted a manageable system that was easy to modify and evaluate regularly. Something that their managers, already comfortable with spreadsheets, could easily adopt, yet sophisticated enough to handle complex statistical modeling. They also wanted a solution that would integrate seamlessly with their ERP system. Finally, they wanted a simple web interface that would allow users to conduct ‘what-if’ scenarios based on multiple factors to arrive at the best possible forecast.
We began by helping them understand their current data – where it lived and how we can best work with it. We worked to break down products around attribute clusters and categories, and looked at customer ordering and production histories to tease out any seasonality, outliers, and other hidden patterns. Finally, we settled on a game plan to develop a custom forecasting solution using the KNIME open-source no-code analytics platform to fully automate the process without licensing costs or the need for custom programming.
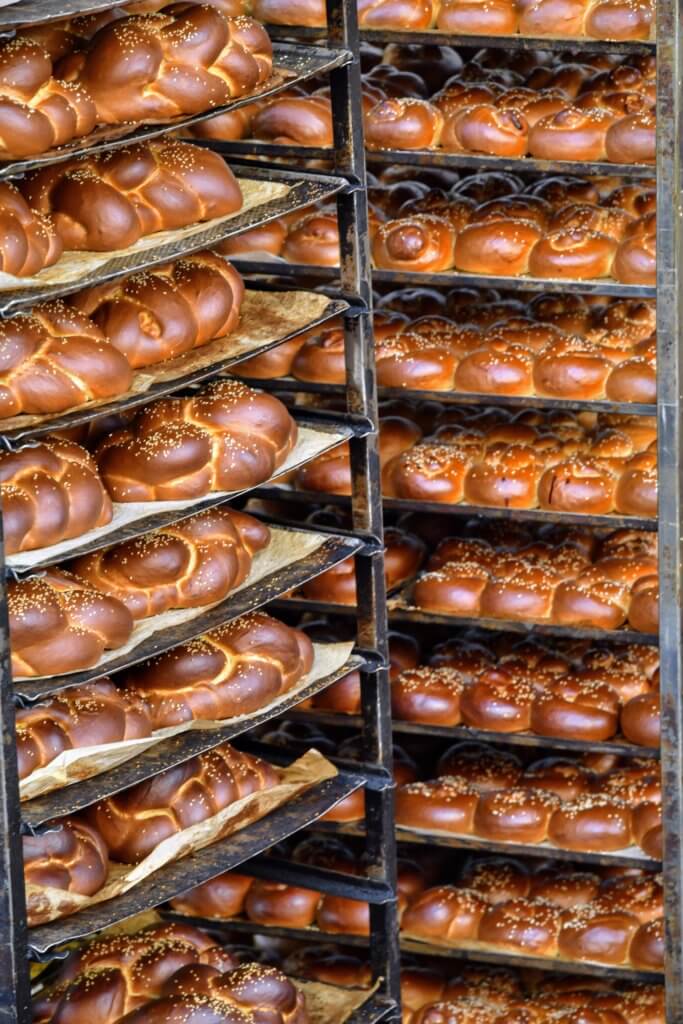
The final forecasting solution was flexible enough to capture qualitative inputs from the field (such as planned promotions by retailers) to adjust forecasts on the fly, and yet robust enough to automate over 75% of their demand planning process.
The final statistical forecast gets translated into Excel and distributed to regional and production managers every week, where they can review projections and plan their runs with confidence. Our customer is so confident with their new projections, in fact, that they often share forecasts with their vendors and are planning to develop a managed inventory program with their larger suppliers as a result.
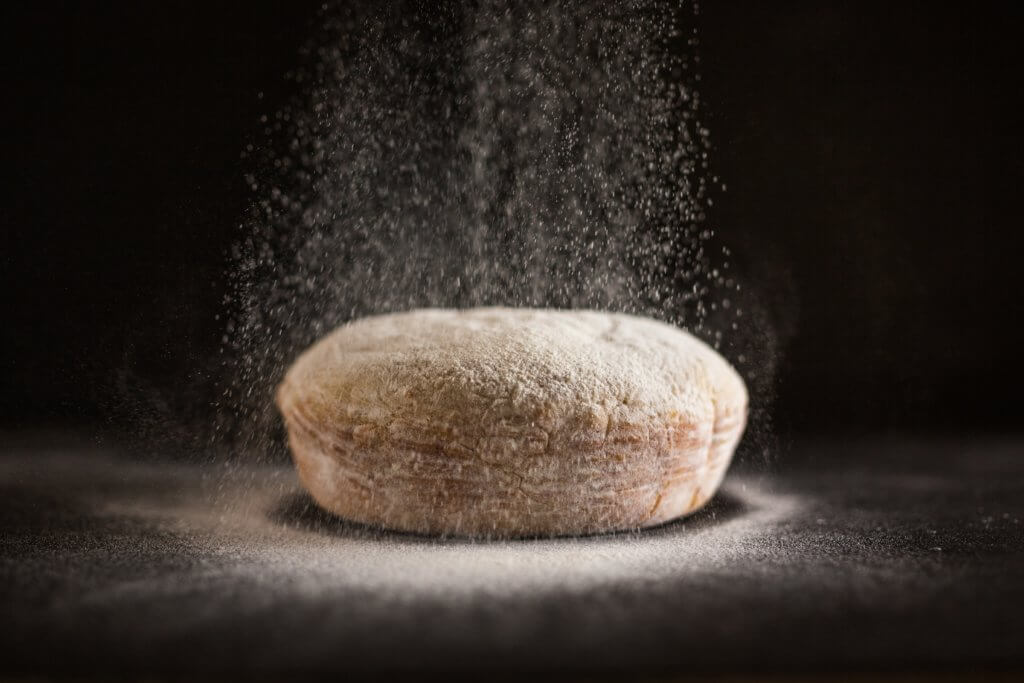
The Many Benefits of Demand Forecasting
Photo by Patrick Fore on Unsplash
The Benefits…
How Leveraging Historical Data Can Benefit The Future of Your Food Business.
With so much hinging on the accuracy of demand forecasts, it’s obvious then that their impact reaches far beyond lost sales and spoiled baked goods.
For example, reliable forecasts can help finance teams accurately estimate costs, profits, and capital requirements for the business; sales departments gain deeper customer insights from projected order volumes; operations teams can better manage labor, supplies, and logistics.
Other benefits from accurately forecasting demand for food products include,
- Better prediction of cash flows.
- More control of operating costs.
- Precise product pricing.
- Improved order fulfillment & turnaround.
- Significant savings on packaging costs (since packaging is SKU-dependent for baked goods).
- More successful product launches.
- Reductions on safety stock by 10-20%.
For commercial bakers, these benefits are particularly important. Packaging is one area that can quickly eat into margins because many baked goods are packaged differently. The ability to forecast demand at the SKU level has had the added benefit of reducing packaging costs associated with overstocks.
More successful new product introductions, and a significant reduction in safety stock meant that investments made toward their forecasting application soon paid for itself many times over.
Just one year after implementation, our customer saw a 16% reduction in finished goods inventory, a 3% increase in order fulfillment, and a 20% reduction in forecasting errors.
With so much to be gained, are you ready to begin your no-code demand forecast journey with us?
What has been your biggest challenge with forecasting demand for your food products?

➼ Are you going to IBIE in September? If so, let’s connect! Reach out to me on LinkedIn: